Admin مدير المنتدى
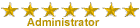
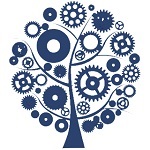
عدد المساهمات : 18984 التقييم : 35458 تاريخ التسجيل : 01/07/2009 الدولة : مصر العمل : مدير منتدى هندسة الإنتاج والتصميم الميكانيكى
 | موضوع: كتاب Artificial Intelligence Techniques for Networked Manufacturing Enterprises Management السبت 30 ديسمبر 2023, 11:01 am | |
| 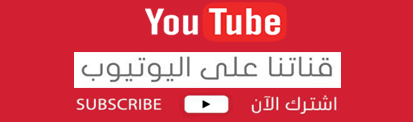
أخواني في الله أحضرت لكم كتاب Artificial Intelligence Techniques for Networked Manufacturing Enterprises Management Lyes Benyoucef , Bernard Grabot Editors
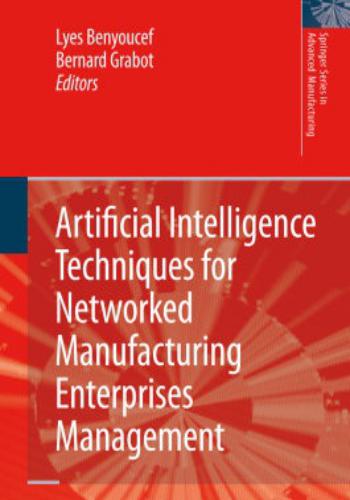 و المحتوى كما يلي :
Contents 1 Intelligent Manufacturing Systems E. Oztemel .1 1.1 Introduction .1 1.2 Traditional Manufacturing Systems 3 1.3 Changes in Manufacturing Systems: a Historical Perspective 6 1.4 Artificial Intelligence and Intelligent Manufacturing Systems .13 1.4.1 Technologies of Artificial Intelligence 13 1.4.2 Intelligent Manufacturing Systems 22 1.5 Properties of Intelligent Manufacturing Systems 25 1.6 Architecture of Intelligent Manufacturing Systems 27 1.7 Holonic Manufacturing Systems .33 1.8 Applications of Intelligent Manufacturing Systems 37 1.9 Conclusions .39 References 39 2 Agent-based System for Knowledge Acquisition and Management Within a Networked Enterprise A.J. Soroka 43 2.1 Agent-based and Related Systems 43 2.1.1 Origins of Agent Research 44 2.1.2 Definition of an Agent .46 2.1.3 Agent Architectures .47 2.1.4 Agent Types and Applications 50 2.1.5 Machine Learning for Generation of Knowledge Bases .53 2.2 Product Fault Knowledge Acquisition and Management .57 2.2.1 Automating Knowledge Base management .57 2.2.2 Analysis of Knowledge Base Management Process 58 2.3 Agent System for Knowledge Acquisition and Management .63 2.3.1 User Agent .65 2.3.2 Server Agent 70xvi Contents 2.3.3 Testing . 79 2.4 Conclusions 81 References . 81 3 Multi-agent Simulation-based Decision Support System and Application in Networked Manufacturing Enterprises H. Ding, W. Wang, M. Qiu and J. Dong . 87 3.1 Introduction 87 3.2 Literature Review . 88 3.2.1 Simulation Methods 89 3.2.2 Multi-agent Simulation . 89 3.2.3 Tools and Applications 90 3.3. Problem Description and Approach . 91 3.3.1 Platform Architecture 92 3.3.2 Multi-agent Supply Chain Simulation Model . 93 3.4 Modeling and Analysis . 94 3.4.1 Baseline Simulation Model . 95 3.4.2 Scenario Analysis 95 3.4.3 Inventory Control Policy . 96 3.4.4 Forecast Accuracy . 97 3.4.5 Build-to-plan vs. Build-to-order 99 3.4.6 Procurement Policy . 100 3.4.7 Truck Utilization . 101 3.5 Conclusions and Perspectives . 103 References . 104 4 A Collaborative Decision-making Approach for Supply Chain Based on a Multi-agent System Y. Ouzrout, A. Bouras, E.-H. Nfaoui and O. El Beqqali . 107 4.1 Introduction 108 4.2 Distributed Simulation and Supply Chain Management . 110 4.2.1 Decision-making and Multi-agent Approaches . 111 4.2.2 Multi-agent Systems Simulation . 111 4.3 The Supply Chain Modeling . 112 4.3.1 Supply Chain Modeling Methodology 113 4.3.2 The Safety Inventory Case Study 114 4.4 The Multi-agent Architecture . 116 4.4.1 The Modeling Principle . 116 4.4.2 Architecture . 117 4.4.3 Negotiation Protocols 120 4.5 Industrial Case Study 122 4.6 Conclusion and Perspectives 125 References . 126Contents xvii 5 Web-services-based e-Collaborative Framework to Provide Production Control with Effective Outsourcing A. Keshari, M. K. Tiwari and R. Teti .129 5.1 Introduction .130 5.2 Literature Review .131 5.3 Design of Web-services-based e-Collaborative Framework .133 5.3.1 The Proposed Framework 133 5.3.2 Design of System Components of Model 134 5.3.3 The Mathematical Model 139 5.3.4 Overall Objective Function .142 5.4 Protocols of Functional Agents .143 5.4.1 Machine Agent Protocol 143 5.4.2 Production Controlling Agent Protocol .144 5.4.3 Task Management Agent (TMA) Protocol 145 5.4.4 Hierarchy of Steps in Web-services-based e-Collaborative System145 5.5 Results and Discussion .145 5.5.1 Sample-sort Simulated Annealing Algorithm .148 5.5.2 Experimental Analysis 148 5.6 Conclusions .157 References 158 6 Isoarchic and Multi-criteria Control of Supply Chain Network F. Ounnar and P. Pujo 161 6.1 Introduction .161 6.2 Supply Chain Management Limits .163 6.3 Control of a Dynamic Logistic Network: Isoarchic and Multi-criteria Control .164 6.3.1 Description of the Interacting Entities .165 6.3.2 Definition of Self-organized Control .166 6.3.3 Support Structure: Autonomous Control Entity 171 6.4 Distributed Simulation of a Dynamic Logistic Network 174 6.4.1 High-level Architecture Components 174 6.4.2 Integration of the DEVS-ACE Models in High-level Architecture Environment 175 6.5 Experiments via Simulation 175 6.6 Conclusion and Future Work 178 References 179 7 Supply Chain Management Under Uncertainties: Lot-sizing and Scheduling Rules A. Dolgui, F. Grimaud and K. Shchamialiova 181 7.1 Introduction .181 7.2 Short Presentation of Lot-sizing and Scheduling Problems 184 7.2.1 Basic Models and Extensions 187xviii Contents 7.2.2 Lot-sizing and Scheduling Under Uncertainties 191 7.2.3 Optimization Techniques 196 7.3 A Case Study 197 7.3.1 Description of the Case Study . 197 7.3.2 Representation of Uncertainties 200 7.3.3 Objective Function 202 7.3.4 Decomposition for Optimization . 203 7.3.5 Numerical Example . 210 7.3.6 Numerical Results . 214 7.4 Conclusions 216 References . 217 8 Meta-heuristics for Real-time Routing Selection in Flexible Manufacturing Systems M. Souier, A. Hassam and Z. Sari 221 8.1 Introduction 222 8.2 Literature Review . 223 8.3 Job-shop 225 8.3.1 Job-shop Problem 225 8.3.2 Simulation of a Flexible Manufacturing System Environment . 226 8.4 Dissimilarity Maximization Method and Modified DMM Rules . 228 8.4.1 Dissimilarity Maximization Method for Real-time Routing Selection 228 8.4.2 Modified Dissimilarity Maximization Method for Real-time Routing Selection 229 8.5 Meta-heuristics for Job-shop Routing . 230 8.5.1 Ant Colony Optimization 230 8.5.2 Simulated Annealing . 231 8.5.3 Particle Swarms Optimization . 232 8.5.4 Genetic Algorithms . 234 8.5.5 Taboo Search . 235 8.5.6 Electromagnetism-like Method . 236 8.6 Performance Evaluation of Routing Selection Methods . 237 8.6.1 System Simulation Without Presence of Breakdown 237 8.6.2 System Simulation With Presence of Breakdown . 241 8.7 Conclusions 246 References . 247 9 Meta-heuristic Approaches for Multi-objective Simulation-based Optimization in Supply Chain Inventory Management D. Sánchez, L. Amodeo and C. Prins 249 9.1 Introduction 249 9.2 Literature Review . 251 9.3 Problem Formulation 253Contents xix 9.4 Implementation of Selected Evolutionary Algorithms 256 9.4.1 Non-dominated Sorting Genetic Algorithm II .256 9.4.2 Strength Pareto Evolutionary Algorithm II 258 9.4.3 Strength Pareto Evolutionary Algorithm IIb 259 9.4.4 Multi-objective Particle Swarm Optimization .260 9.5 Computational Experiments and Analysis 262 9.5.1 Evaluation Criteria 262 9.5.2 Parameters Used 263 9.5.3 Experimental Results .263 9.6 Conclusion 267 References 268 10 Diverse Risk/Cost Balancing Strategies for Flexible Tool Management in a Supply Network D. D’Addona and R. Teti 271 10.1 Introduction .271 10.2 Multi-agent Tool Management System 272 10.3 Flexible Tool Management Strategies 275 10.3.1 Current Inventory-Level Decision Making (INVADAPT) .277 10.3.2 Fixed-Horizon Inventory-Level Decision Making (I-FUTURE) 279 10.3.3 Variable-Horizon Inventory-Level Decision Making (INVADAPT_NS) .280 10.4 Tool Inventory Management Simulations 281 10.4.1 Simulation Through INVADAPT .287 10.4.2 Simulation Through I-FUTURE .294 10.4.3 Simulation Through INVADAPT_NS 301 10.5 Test Case Applications .309 10.6 Conclusions and Future Work 312 References 312 11 Intelligent Integrated Maintenance Policies for Manufacturing Systems N. Rezg and S. Dellagi 315 11.1 Introduction .315 11.2 Optimal Maintenance Policy Considering the Influence of the Production Plan on the Deterioration of the Manufacturing System 318 11.2.1 Problem Statement 318 11.2.2 Problem Formulation .319 11.2.3 Influence of Manufacturing System Deterioration on the Optimal Production Plan .324 11.2.4 Optimization of the Maintenance Policy .326 11.3 Intelligent Periodic Preventive Maintenance Policy in Finite Horizon with an Adaptive Failure Law 330 11.3.1 Problem Description 330xx Contents 11.3.2 Model Formulation and Intelligent Determination of the Optimal Solution . 332 11.3.3 Numerical Example . 335 11.4 Conclusions 338 References . 339 12 Enhancing the Effectiveness of Multi-pass Scheduling Through Optimization via Simulation T. Yoo, H.-B. Cho and E. Yücesan 341 12.1 Introduction 342 12.2 Multi-pass Scheduling Using Nested Partitions and Optimal Computing Budget Allocation . 346 12.2.1 The Proposed Multi-pass Scheduling Framework . 346 12.2.2 The Outer Loop: Nested Partitions 346 12.2.3 The Inner Loop: Optimal Computing Budget Allocation . 348 12.3 Implementation of Nested Partitions and Optimal Computing Budget Allocation 349 12.3.1 Nested Partitions: Partitioning Strategy 349 12.3.2 Nested Partitions: Sampling Strategy 351 12.3.3 Nested Partitions: Backtracking Strategy 351 12.3.4 Optimal Computing Budget Allocation: Ranking and Selection Strategy . 352 12.3.5 Performance of Nested Partitions and Optimal Computing Budget Allocation 353 12.4 Experimental Design and Analysis . 354 12.4.1 Experimental Assumptions 354 12.4.2 Experimental Design . 356 12.4.3 Experimental Results for the Probability of Correct Selection 357 12.5 Conclusions 363 References . 364 13 Intelligent Techniques for Safety Stock Optimization in Networked Manufacturing Systems B. Desmet, E.-H. Aghezzaf and H. Vanmaele . 367 13.1 Introduction 367 13.2 Multi-echelon Inventory Control in Networked Manufacturing Systems 369 13.3 Literature Review . 374 13.4 System Safety Stock Optimization in n-echelon Distribution Systems 377 13.4.1 Introduction of a Two-echelon Distribution System . 378 13.4.2 Characterization of the Back-order Service Time in the Central Warehouse . 380 13.4.3 Characterization of the Actual Retailer Replenishment Lead Time . 382Contents xxi 13.4.4 System Safety Stock Optimization in a Two-echelon Distribution System .384 13.5 System Safety Stock Optimization in n-echelon Assembly Systems 386 13.5.1 Introduction of a Two-echelon Assembly System .386 13.5.2 Characterization of the Backorder Service Time for a Subset of Components .389 13.5.3 Characterization of the Incoming Service Time to the Assembly .390 13.5.4 Characterization of the Actual Assembly Lead Time .391 13.5.5 System Safety Stock Optimization in a two-echelon Assembly System .392 13.5.6 Distribution System as Special Case of the Assembly System 394 13.6 System Safety Stock Optimization in Generic Networks .395 13.6.1 Introduction of a Spanning Tree System .395 13.6.2 Characterization of Actual Replenishment Lead Times in a Spanning Tree System .402 13.6.3 System Safety Stock Optimization for a Spanning Tree System .415 13.6.4 Extension to Generic Systems .416 13.7 Conclusions .418 References 419 14 Real-world Service Interaction with Enterprise Systems in Dynamic Manufacturing Environments S. Karnouskos, D. Savio, P. Spiess, D. Guinard, V. Trifa and O. Baecker .423 14.1 Motivation 424 14.2 Real-world Awareness .426 14.2.1 Device Integration Protocols 426 14.2.2 Device-to-Business Coupling .428 14.2.3 Integrating Heterogeneous Devices 429 14.3 Enterprise Integration 430 14.4 Integrating Manufacturing Equipment with the SOCRADES Integration Architecture .432 14.5 Towards Dynamic Adaptation .435 14.5.1 Simulation 437 14.5.2 Self-healing Mechanisms .438 14.5.3 Self-optimizing Mechanisms 439 14.6 Concept Validation in Prototypes 440 14.6.1 Machine Monitoring, Dynamic Decision and Order Adaptation 441 14.6.2 The Future Shop Floor: Mashup of Heterogeneous Service-orientedarchitecture Devices and Services .444 14.6.3 Dynamic Supply Chain Management Adaptation 446 14.6.4 Taming Protocol Heterogeneity for Enterprise Services 449 14.6.5 Energy Monitoring and Control via Representational State Transfer 451 14.7 Discussion and Future Directions 454xxii Contents 14.8 Conclusions and Future Work . 455 References . 455 15 Factory of the Future: A Service-oriented System of Modular, Dynamic Reconfigurable and Collaborative Systems A.-W. Colombo, S. Karnouskos and J.-M. Mendes . 459 15.1 Introduction 460 15.2 The Emergence of Cooperating Objects . 461 15.3 The Cross-layer Service-oriented-architecture-driven Shop Floor . 463 15.4 Dynamic Reconfiguration of a Service-oriented-architecture-based Collaborative Shop Floor . 465 15.4.1 Methodology . 466 15.4.2 Example . 468 15.5 Analysis Behind the Engineering Methods and Tools 469 15.5.1 Applying Functional Analysis to Validate Service Composition Paths in High-level Petri-net-based Orchestration Models . 469 15.5.2 Example . 472 15.6 A Service-oriented-architecture-based Collaborative Production Management and Control System Engineering Application 474 15.7 Conclusions and Future Work 479 References . 480 16 A Service-oriented Shop Floor to Support Collaboration in Manufacturing Networks J. Barata, L. Ribeiro and A.-W. Colombo . 483 16.1 Introduction 483 16.2 Agility in Manufacturing 485 16.3 Collaborative Networks 487 16.4 Service-oriented-architecture as a Method to Support Agility and Collaboration . 488 16.5 Architecture 493 16.5.1 The Role of Componentization . 493 16.5.2 Service Exposure, Composition and Aggregation . 495 16.5.3 The Role of Orchestration in Control, Monitoring and Diagnosis 496 16.6 An Implementation . 498 16.6.1 Manufacturing Device Service Implementation 498 16.6.2 Coalition Leader Service Implementation . 498 16.6.3 Application Example: a Collaborative Pick-and-place Operation. 499 16.7 Conclusions 501 References . 501 Index.……………………………………………………………………………505 Index A Agent-based system, 41, 43, 126 Ant colony optimization, 221 Artificial intelligence, 1, 11, 13, 14, 21, 22, 43−45, 81, 83−85, 126, 131, 484 Assembly system, 196, 218−220, 367, 374−377, 386, 388, 389, 393−395, 403, 418, 419, 477, 479, 502 Availability, 114, 132, 139, 141, 145, 148− 152, 156, 157, 219, 222, 284, 285, 297, 315, 316, 318, 335− 339, 433, 490 B Budget allocation, 341, 345, 354, 364 Business processes, 52, 58, 63, 83, 423, 425, 426, 430, 431, 439 C Capacitated lot-sizing problem, 190 Collaboration, 90, 107, 108, 113, 114, 116, 129, 130, 133, 136, 272, 459− 463, 466, 469, 475, 477, 479, 483, 484, 488, 494, 501 Collaborative system, 459, 463, 479 D Decision-making, 2, 13, 58, 73, 75, 88, 90, 91, 104, 107, 110−112, 119−121, 125, 133, 161, 162, 164−166, 169, 171, 182, 221, 223, 247, 341, 342, 356, 363 Device integration, 427, 455, 464 Device profile for web services (DPWS), 425, 427, 428, 432, 448, 451, 454, 478, 484, 490, 501, 502 Distributed simulation, 89, 126, 161, 162, 174 Distributed system, 126 Distribution system, 90, 367, 368, 375, 377−380, 385, 386, 392, 394, 395, 418−420 Dynamic manufacturing, 426 Dynamic reconfigurable system, 459 E e-collaboration, 129, 133 Economic lot scheduling problem, 189 Economic order quantity, 187 Economic production quantity, 188 Electromagnetic meta-heuristic, 221 Energy monitoring, 451 Enterprise integration, 158, 423, 430 Enterprise resource planning (ERP), 108, 426, 464, 466, 477, 478 Enterprise system, 424−426, 429, 436, 444, 449, 454, 456, 459, 463, 466, 477, 479, 481 Evolutionary algorithm, 222, 249, 250, 252, 256, 264, 269 Expert system, 15, 16, 37, 44, 58, 73, 342, 343506 Index F Failure rate, 315, 318, 319, 323−325, 327, 339 Flexible manufacturing system, 1, 132, 159, 221, 222, 226, 248, 364, 365, 485 Flow-shop, 182, 183, 185−187, 191, 198, 217, 218, 340 Fuzzy logic, 14, 19, 20, 38, 365 G Generic networked manufacturing system, 367 Genetic algorithm, 14, 18, 19, 37, 39, , 111, 125, 158, 181, 184, 197, 205, 207, 216, 217, 219, 220, 223, 225, 234, 246, 252, 253, 256, 268, 269, 342−344, 365 Graph, 344, 370, 372, 392, 395, 417, 441, 453 H Holonic, 1, 33, 36, 40, 161, 162, 164, 165, 172, 178, 180 I Intelligent agent, 45, 46, 83−85, 120, 271, 272, 274, 312, Internet of things, 423, 424, 428, 440, 456, 462 Inventory control, 92, 94, 96, 97, 218, 277, 312, 367, 368, 370, 371, 373, 374, 419 Isoarchic control model, 161 J Job-shop, 183, 185−187, 223−226, 246, 247 K Knowledge acquisition, 53 Knowledge management, 61, 127, 465 L Lead time, 191−194, 201, 217−219 Lot-sizing, 181−184, 187, 191, 195, 196, 197, 217, 218, 220, 373, 374, 419, 420 M Machine learning, 43, 57, 61, 81−83, 85 Maintenance, 315−319, 322, 324, 326, 329− 332, 334−340 Manufacturing network, 91, 434, 483, 484, 488, 489, 492 Manufacturing system, 1−3, 6−8, 10, 11, 13, 14, 22−27, 29, 33, 36−41, 44, 165, 180, 182, 183, 191, 251, 316, 318, 319, 338−340, 344, 485, 502 Material requirement planning, 192 Meta-heuristic, 221−226, 230, 236, 237, 239−243, 245−247, 249 Middleware, 424, 426, 432, 433, 446, 502 Multi-agent, 45, 52, 83, 84, 87−91, 103, 105, 107, 111, 116, 126, 129, 130, 132, 133, 158, 179, 271, 272, 273, 313, 315, 491, 501 Multi-criteria, 161, 162, 164, 166, 168−170, 269 Multi-objective optimization, 249 Multi-pass scheduling, 341−343, 345−349, 351, 354, 356−358, 363, 364 N Nested partitions, 345, 365 Networked enterprise, 43, 177 Networked manufacturing enterprise, 87 Networked manufacturing system, 367, 368, 370 Neural network, 75, 342, 344, 365, 367 O OPC-UA, 425, 450 Open-shop, 185, 187 Optimization, 126, 148, 162, 164, 166, 168, 172, 179, 180, 181, 189, 196, 200, 204, 205, 215, 217, 218, 219, 221, 222, 224, 231−Index 507 233, 247−254, 256, 262, 268, 269, 316, 318, 326, 329, 339, 341, 345, 364, 365, 367, 368, 372, 374−377, 385, 386, 393, 405, 415, 417−420, 422, 423, 434, 436, 439, 446, 454, 493 Outsourcing, 129, 130−132, 134, 138, 142, 145, 148, 156−158, 419 P Parallel machines, 185 Planning horizon, 184, 185, 187, 189, 190, 197−199, 374 Production control, 158, 315, 316, 465, 476, 479 Production system, 184, 185, 193, 216, 220, 222−224, 227, 229, 237, 246, 316, 420, 459, 463, 465, 470, 474, 478, 480, 481, 486, 501 R Random yield, 183, 194, 195, 201, 217, 219 Real-time routing, 221, 228, 229 Real-time scheduling, 222−224, 341, 342, 365 Reliability, 169, 318, 319, 332, 338, 339, 433, 455 Renewal process, 193, 201, 218 Representational state transfer (REST), 425, 427, 450, 451, 453, 456, 457 Radio frequency identification (RFID), 432, 444, 446, 456 S Safety stock, 367, 375, 418, 419 SAP, 423, 424, 432, 433, 439, 441−443, 445, 446, 448, 449, 459, 461, 478 Scheduling, 51, 83, 91, 94, 105, 112, 127, 129, 130−132, 138, 139, 145, 148, 157−159, 166, 179, 181, 184, 221−226, 246−248, 339, 341−345, 348, 349, 356, 363−365, 423, 446 Sequencing, 132, 158, 181−184, 197, 216− 218, 220, 223, 225, 344, 365 Service interaction, 423 Service-oriented architecture, 424, 428, 444, 459, 461, 463−465, 470−473, 477, 479−481, 489, 501 Service-oriented device, 503 Service-oriented ecosystem, 490 Service-oriented infrastructure, 484 Service-oriented modelling, 491 Service-oriented shop floor, 483 Service-oriented paradigm, 456 Simulated annealing, 148, 157−159, 223, 225, 231, 246, 247, 252, 342, 344, 364 Simulation, 87−97, 99, 101−105, 110−112, 126, 127, 149−151, 161, 174− 177, 179−181, 184, 214, 217, 226, 246−254, 263, 268, 271, 272, 281, 283, 286−288, 295, 299, 301, 302, 307, 312, 314, 339, 341, 342, 344−349, 352−357, 359, 363−365, 369, 374, 376, 383−386, 391, 393, 394, 402, 415−418, 421, 423, 426, 435−438, 446, 455, 456 Single machine, 183, 185, 197, 201, 219 SOCRADES, 424, 430, 455, 463, 471, 473, 475, 477−480, 490, 503 Supply chain, 88−95, 99, 100, 104, 105, 107−115, 117, 119, 122, 125− 127, 132, 158, 161−163, 179−182, 218, 226, 250−254, 256, 260, 268, 269, 312, 313, 372, 374, 375, 398, 400−415, 418−420, 422, 423, 426, 434, 480, 485−488, 501, 502 Supply network, 271−273, 275, 313 Swarm optimization, 256 T Taboo, 221, 222, 235 Tool management, 223, 271, 272, 275, 312, 313508 Index U Uncertainty, 107, 111, 114, 130, 181, 191− 196, 218−220, 370, 373, 420, 485 W Wagner–Whitin, 189 Web-oriented querying interface, 429 Web-service, 129, 130, 133, 462, 475, 478, 481
كلمة سر فك الضغط : books-world.net The Unzip Password : books-world.net أتمنى أن تستفيدوا من محتوى الموضوع وأن ينال إعجابكم رابط من موقع عالم الكتب لتنزيل كتاب Artificial Intelligence Techniques for Networked Manufacturing Enterprises Management رابط مباشر لتنزيل كتاب Artificial Intelligence Techniques for Networked Manufacturing Enterprises Management 
|
|