Admin مدير المنتدى
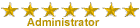
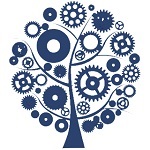
عدد المساهمات : 18745 التقييم : 34763 تاريخ التسجيل : 01/07/2009 الدولة : مصر العمل : مدير منتدى هندسة الإنتاج والتصميم الميكانيكى
 | موضوع: كتاب Effective Resource Management in Manufacturing Systems - Optimization Algorithms for Production Planning الثلاثاء 12 سبتمبر 2023, 1:57 am | |
| 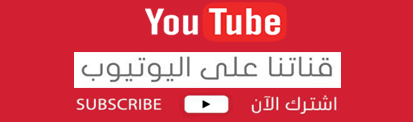
أخواني في الله أحضرت لكم كتاب Effective Resource Management in Manufacturing Systems - Optimization Algorithms for Production Planning Massimiliano Caramia and Paolo Dell’Olmo
 و المحتوى كما يلي :
Contents List of Figures . xv List of Tables xix 1 Manufacturing Systems: Trends, Classification, and Behavior Patterns . 1 1.1 Distributed Flexible Manufacturing Systems 2 1.1.1 DFMS Properties . 4 1.1.2 DFMS Behaviour 7 1.1.3 Organizational Paradigms 8 1.1.4 Example of the Implementation of a Holonic Manufacturing System in Induction Motor Production . 10 1.1.5 A Layered Approach to DFMS Modeling . 13 1.2 Manufacturing Control (MC) . 14 1.2.1 Definition of Manufacturing Control . 14 1.2.2 Manufacturing Control Functions 16 1.2.3 Classification of Production Scheduling . 18 1.3 Scheduling and Resource Allocation . 23 1.3.1 Definition 23 1.3.2 Mathematical Model for Job Shop Scheduling . 24 1.4 On-line Manufacturing Control . 27 1.4.1 Computational Complexity of Scheduling and Resource Allocation . 28 1.5 Algorithmic Approach to Problem Solution . 28 1.5.1 Greedy Heuristics . 29 1.5.2 Local Search Heuristics 30 1.5.3 Off-line and On-line Algorithms 32 1.6 Conclusions . 33 xixii Contents 2 On-Line Load Balancing 35 2.1 Problem Definition 35 2.2 Known Results and Existing Approaches . 36 2.2.1 The Greedy Approach . 37 2.2.2 The Robin-Hood Algorithm . 39 2.2.3 Tasks with Known Duration: the Assign1 Algorithm . 41 2.3 A Metaheuristic Approach 43 2.4 Example . 48 2.5 Experimental Results 53 2.5.1 A Multi-objective Approach in the Case of Known Task Departure Dates 57 2.6 Conclusions . 62 3 Resource Levelling . 65 3.1 Background and Problem Definition . 65 3.2 Resource Levelling and the Minimization of the Peak and the Makespan 68 3.3 The Greedy Approach . 75 3.4 The Metaheuristic Approach 78 3.4.1 Conceptual Comparison with Known Local Search Methods . 79 3.4.2 How to Control the Effect of the Minimization of the Makespan and the Frequency Based Memory . 82 3.5 Experimental Results 84 3.5.1 Description of the Experiments 84 3.5.2 Analysis of the Results . 85 3.5.3 Lower Bounds Comparison . 87 3.5.4 Comparison with Known Algorithms . 90 3.6 The Extension to the Case with Arbitrary Integer Duration . 93 3.7 Case Study 1 . 95 3.8 Case Study 2 . 100 3.9 Conclusions . 103 4 Scheduling Jobs in Robotized Cells with Multiple Shared Resources . 105 4.1 Background and Problem Definition . 105 4.2 Problem Definition 107 4.3 N P-Completeness Result . 109 4.4 The Proposed Heuristic 110 4.5 Computational Results . 114 4.6 Conclusions . 120Contents xiii 5 Tool Management on Flexible Machines 121 5.1 Background . 121 5.1.1 Definition of the Generic Instance . 126 5.1.2 Assumptions 126 5.2 The Binary Clustering and the KTNS Approaches . 127 5.3 The Proposed Algorithms 130 5.3.1 Algorithm 1 130 5.3.2 Algorithm 2 135 5.3.3 Algorithm 3 141 5.3.4 Algorithm 4 148 5.4 Computational Analysis 153 5.4.1 Comparison with Tang and Denardo 153 5.4.2 Comparison with Crama et al . 155 5.4.3 Comparison Among the Proposed Algorithms . 163 5.5 Conclusions . 168 Appendix A . 171 Appendix B . 193 Glossary . 199 References . 201 Index 215 List of Figures 1.1 Example of a Virtual Enterprise. The Virtual Enterprise is a temporary network of independent companies linked by information technology to share skill, costs and access to one another markets 2 1.2 The Extended Enterprise 3 1.3 The Supply Chain . 4 1.4 Configuration of an autonomous distributed manufacturing system 5 1.5 Production methods 11 1.6 Basic holons . 12 1.7 A shop production floor . 14 1.8 Conventional manufacturing control system 15 1.9 Holonic manufacturing control system 16 1.10 Configuration of a decentralized control system . 17 1.11 Manufacturing control functions 17 1.12 Example of a flow shop with four machines and two jobs 19 1.13 An assembly line . 20 1.14 Example of a schedule in a flow shop . 20 1.15 Example of a job shop with four machines and three jobs 21 1.16 Example of a schedule in a job shop 21 1.17 Example of a fixed position shop . 22 1.18 Example of precedence graph 26 1.19 Schedule of the precedence graph in Figure 1.18 on two resources 26 1.20 Greedy schedule for MTSP 30 1.21 Schedule for MTSP obtained by local search . 31 1.22 On-line schedule for MTSP 32 1.23 Off-line schedule for MTSP 33 xvxvi List of Figures 2.1 The trend of the objective function of the greedy algorithm over time: the instance with 100 tasks and 5 machines 55 2.2 The trend of the objective function of the semi-greedy algorithm 56 2.3 Schedule shape produced by the greedy algorithm on an instance with 200 tasks and 10 machines 58 2.4 Schedule shape produced by the OBA-RH revised algorithm on an instance with 200 tasks and 10 machines . 59 2.5 Schedule shape produced by the greedy algorithm on an instance with 500 tasks and 10 machines 59 2.6 Schedule shape produced by the OBA-RH revised algorithm on an instance with 500 tasks and 10 machines . 60 2.7 Load shape of machine 1 over time interval [t, t + 3] . 60 2.8 Load shape of machine 2 over time interval [t, t + 3] . 61 2.9 Load shape of machine 3 over time interval [t, t + 3] . 61 2.10 Tuning of the multi-objective parameters 62 3.1 The incompatibility graph . 73 3.2 The resource requirement matrix . 74 3.3 The trade off between the schedule length and the peak of resource usage. The notation i(q) in the schedules means that task i requires q units of the associated resource type. By default i = i(1) . 74 3.4 The trade off between the schedule length and the peak of resource usage. The notation i(q) in the schedules means that task i requires q units of the associated resource type. By default i = i(1) . 74 3.5 Schedule obtained by means of STRF . 77 3.6 Schedule obtained by means of LTRF . 77 3.7 Schedule obtained by means of ATR 78 3.8 The multi-start greedy algorithm structure. Parameter count is used to check if the local search in the hill climbing phase is executed more than once 81 3.9 The experiments are averages of 10 instances. The x-axis reports the value α; + are the lowest values of the makespan and * the highest ones 83 3.10 Up: the chart shows the sequence of dev obtained by the algorithm in the scenario B1 and δ = 8. In the x-axis are the number of progressive invocation of the algorithm functions. Down: the chart shows the different dev-makespan combinations explored by the algorithm in 12,500 iterations on the same scenario B1 with δ = 8 . 88 3.11 Production layout for the three antenna types 96List of Figures xvii 3.12 The antenna assembly process . 98 3.13 The graph associated with the production process 100 3.14 The production line . 101 4.1 Robots in a cell 106 4.2 An example of the LMU Problem 109 4.3 An optimal schedule with Y = 2z(B + 2) 110 4.4 An application of the LMUA algorithm . 114 4.5 Trends of the makespan as the number n of jobs increases . 115 4.6 Trends in the makespan as the number m of machines increases 116 4.7 Makespan values, for a given m, as n and pr increase . 117 4.8 Makespan values, for a given n, as m and pr increase . 118 5.1 An NC machine 122 5.2 Example of tools for NC machines . 123 5.3 The compatibility graph . 133 5.4 A matching in the compatibility graph 133 5.5 The new graph G′ 134 5.6 Iterated Step 4 and Step 5 . 134 5.7 Graph of the intersections among configurations 140 5.8 Graph of the intersections among configurations 144 5.9 Graph of the intersections among configurations 145 5.10 Graph of the intersections among configurations 146 5.11 Graph of the intersections among configurations 147 5.12 Graph of the intersections among configurations 148 5.13 Graph of the intersections among configurations 148 5.14 Step 4: graph of the intersections among configurations 149 5.15 Graph of the intersections among configurations 150 5.16 Graph H(V, E) . 151 5.17 First iteration of Step 2 . 151 5.18 Second iteration of Step 2 . 152 5.19 Comparison with Tang and Denardo [164] 155 5.20 Comparison with average values 165 5.21 Average values for dense matrices 167 5.22 Average performance of the algorithms on instances 10/10/7, 15/20/12, 30/40/25, 40/60/30 . 168 5.23 Number of times each algorithm outperforms the others . 169List of Tables 1.1 Basic and characterizing properties of DFMS 5 1.2 Greedy Algorithm for MSTP . 30 1.3 Local search algorithm for MTSP 31 2.1 Metaheuristic template 44 2.2 SA template; max it is a limit on number of iterations 44 2.3 TA template; max it is a limit on the number of iterations . 45 2.4 OBA template; max it is a limit on the number of iterations . 46 2.5 OBA-RH template; max it is a limit on the number of iterations 47 2.6 OBA-RH revised template; max it is a limit on the number of iterations 49 2.7 Comparison among different on-line load balancing algorithms. The number of machines is 5 . 53 2.8 Comparison among different on-line load balancing algorithms. The number of machines is 10 54 2.9 Comparison among different on-line load balancing algorithms. The number of machines is 15 54 2.10 Comparison among different on-line load balancing algorithms. The number of machines is 20 55 2.11 Comparison among different on-line load balancing algorithms. The average case with 5 machines 56 2.12 Comparison among different on-line load balancing algorithms. The average case with 10 machines . 57 2.13 Comparison among different on-line load balancing algorithms. The average case with 15 machines . 57 2.14 Comparison among different on-line load balancing algorithms. The average case with 20 machines . 58 xixxx List of Tables 2.15 Comparison among different on-line load balancing algorithms with a multi-objective approach. The average case with 5 machines 63 2.16 Comparison among different on-line load balancing algorithms with a multi-objective approach. The average case with 10 machines 63 2.17 Comparison among different on-line load balancing algorithms with a multi-objective approach. The average case with 15 machines 64 2.18 Comparison among different on-line load balancing algorithms with a multi-objective approach. The average case with 20 machines 64 3.1 Greedy algorithm (emphasis on makespan) 76 3.2 Greedy algorithm (emphasis on peak) . 77 3.3 Total, maximum and average resource requests in the example of the previous section 77 3.4 The local search algorithm . 80 3.5 The 12 scenarios examined 86 3.6 Experimental results for δ = 2 . 87 3.7 Experimental results for δ = 4 . 89 3.8 Experimental results for δ = 6 . 90 3.9 Experimental results for δ = 8 . 91 3.10 Comparison of Mpeak with LBpeak . 92 3.11 Comparison of Mpeak with LBpeak ′ . 92 3.12 Comparison of Mts with Opt 93 3.13 Comparison between our algorithm and the algorithms in [138] . 93 3.14 Comparison between our algorithm and the algorithms in [138] . 94 3.15 Duration and resources associated with each task of the antenna assembly process. Task duration are in hours . 97 3.16 Precedence relations among tasks . 99 3.17 Duration and resources associated with each task of the antenna assembly process. Task duration are in hours . 101 4.1 Scenario with pr = 0 115 4.2 Scenario with pr = 0.1 116 4.3 Scenario with pr = 0.2 116 4.4 Scenario with pr = 0.3 117 4.5 Scenario with pr = 0.4 117 4.6 Reducing processing times of Make operations . 119 4.7 Percentage reduction of Cmax 119 4.8 Reducing processing times of Load and Unload operations . 119List of Tables xxi 4.9 Percentage reduction of Cmax 119 5.1 A tool/job matrix 128 5.2 The score of each row . 128 5.3 The matrix ordered with respect to the rows . 129 5.4 The scores of each column . 129 5.5 The matrix ordered with respect to the columns 129 5.6 The final matrix ordered 130 5.7 Tool/job matrix 132 5.8 Step 1: first iteration 137 5.9 Step 1: second iteration . 138 5.10 Step 1: third iteration . 138 5.11 Step 1: fourth iteration 139 5.12 Tool/job matrix after Step 2 . 139 5.13 Intersections among configurations 140 5.14 Intersections among configurations 143 5.15 Intersections among configurations 144 5.16 Intersections among configurations 145 5.17 Intersections among configurations 146 5.18 Intersections among configurations 147 5.19 Intersections among configurations 149 5.20 Complete table of removed edges according to the out-degree values . 152 5.21 Matrix obtained in Step 5 . 153 5.22 Performance of the four algorithms proposed . 154 5.23 min-max intervals of each test instance . 156 5.24 The different capacities used in the test instances . 156 5.25 Average values of best(I) 157 5.26 Best results achieved by the four algorithms on the 160 test instances 157 5.27 Best results achieved by the four algorithms on the 160 test instances 158 5.28 Results of Algorithm 1 on the 160 test instances 159 5.29 Results of Algorithm 1 on the 160 test instances 159 5.30 Comparison between Algorithm 1 and the algorithms given in [58] . 160 5.31 Results of Algorithm 2 on the 160 test instances 160 5.32 Results of Algorithm 2 on the 160 test instances 161 5.33 Comparison between Algorithm 2 and the algorithms given in [58] . 161 5.34 Results of Algorithm 3 on the 160 test instances 162 5.35 Results of Algorithm 3 on the 160 test instances 162xxii List of Tables 5.36 Comparison between Algorithm 3 and the algorithms given in [58] . 163 5.37 Results of Algorithm 4 on the 160 test instances 164 5.38 Results of Algorithm 4 on the 160 test instances 164 5.39 Comparison between Algorithm 4 and the algorithms given in [58] . 165 5.40 Comparison among the four algorithms proposed . 166 5.41 Average values for dense matrices 166 5.42 Average performance of the algorithms on instances 10/10/7, 15/20/12, 30/40/25, 40/60/30 . 167 5.43 Number of times an algorithm outperforms the others . 168 Index adaptability, 5–7 agile manufacturing, 6 agility, 5–7 AMPL, 193, 195, 197 Aneja, 106 Asfahl, 106 autonomy, 4, 5, 10 Azar, 36 Belady, 124 binary clustering algorithm, 127 bionic manufacturing, 8 Blazewicz, 106 Braha, 125 Ciobanu, 67 competitive ratio, 36, 37, 40, 41 CPLEX, 193 Crama, 107, 123, 124, 155, 156 decentralization, 5 Denardo, 124, 153, 155, 169 distributed flexible manufacturing systems, 2, 4, 7, 13 distributed manufacturing, 1, 7, 8 distributed manufacturing control, 13 Djelab, 125 dynamism, 5 extended enterprise, 3 Finke, 125 flexibility, 5, 6, 11, 12, 18, 19, 21, 22, 26 flexible machines, 121 flexible manufacturing systems, 6 215216 Index flexible manufacturing systems, 9, 21, 121 flow shop, 19–22, 24, 105, 107 Ford, 1 fractal factory, 8 Graham, 36 GRASP, 79, 80 greedy, 37–39, 44, 53–59, 62, 63, 78– 81, 124, 125 Gronalt, 125 GTNS, 126 Gunther, 125 Hall, 106, 107 Hartley, 107 hill climbing, 43, 81 holon, 9, 10, 12, 13 holonic manufacturing, 8–10, 15, 16 job shop, 1, 19, 21, 22, 24, 107, 121 Kamoun, 106 Koestler, 9 KTNS, 124, 125, 150, 154, 169 Kuo, 126 Levner, 107 Levy, 67 load balancing, 35–37, 41–43, 53–58, 65 Lofgren, 125 Maimon, 125 makespan, 37, 65, 67, 68, 72, 74, 75, 78, 102, 103, 107, 108 mass manufacturing, 1 master schedule, 15 matching, 130, 132, 133 Matzliach, 125 McGinnis, 125 modelon, 9 Moder, 67 MRP, 15 Narendran, 125 numeric control machine, 121, 123, 153 old bachelor acceptance, 45 on-line algorithm, 32, 37, 41 on-line manufacturing control, 16, 18, 27, 28 open hierarchical systems, 8 Phillips, 37, 67 Privault, 125 Rajkurmar, 125 reaction, 5 resource allocation, 12, 16, 18, 23, 25, 27, 28, 35, 65, 67, 71, 82 restricted candidate list, 38, 39, 80 Robin-Hood, 39, 40, 43, 45–50, 53–60, 63 Rupe, 126 Sadiq, 125 semi-greedy, 38, 53–58 Simon, 9 simulated annealing, 43–45 Slow-Fit, 38 Stecke, 121 supply chain, 3, 4 Tang, 124, 153, 155, 169 threshold acceptance, 43–45 tool switching, 123–125, 127, 132, 135 TSP, 125 Tzur, 125 Van De Klundert, 107 virtual enterprise, 1, 4 Warneke, 9 Westbrook, 37 Wiest, 67 Wilhelm,
كلمة سر فك الضغط : books-world.net The Unzip Password : books-world.net أتمنى أن تستفيدوا من محتوى الموضوع وأن ينال إعجابكم رابط من موقع عالم الكتب لتنزيل كتاب Effective Resource Management in Manufacturing Systems - Optimization Algorithms for Production Planning رابط مباشر لتنزيل كتاب Effective Resource Management in Manufacturing Systems - Optimization Algorithms for Production Planning 
|
|